Frank A. Cowell and Emmanuel Flachaire, Quantitative Economics, 2018, 9, 865-901.
Emmanuel Flachaire is a Professor of Economics at Aix-Marseille University. He received his PhD in 1998 from the Université de la Méditerranée. His fields of interest include econometrics, machine learning, and the measurement of inequality and mobility.
The research program
Mobility is an important concept in several branches of social science and economics. However, convincing evidence of mobility requires not only good data but also measurement tools that have appropriate properties. Perhaps surprisingly, several commonly-used techniques and indices do not appear to conform well to simple principles concerning mobility and immobility. As an example, consider the commonly-used measure of mobility 1−β, where β is an elasticity coefficient, computed as the ordinary least-squares estimation of the slope coefficient from a linear regression of log-income in period 1 on log-income in period 0. It has been used in almost every empirical study of intergenerational income mobility. However, this index has a major drawback. While a large value of 1−β may provide evidence of significant mobility, a low value does not necessarily imply low mobility. To see why, take three people with log-incomes equal to, respectively, (1,1.5,2) in period 0 and (1,3,2) in period 1. In this case, the index 1−β is equal to zero, suggesting there is no mobility even though there is clear evidence of income mobility (the second person’s log-income doubles, while the others remain unchanged). This example shows that the elasticity-based index is inadequate to measure income mobility; it illustrates the need to develop mobility measures with appropriate properties.
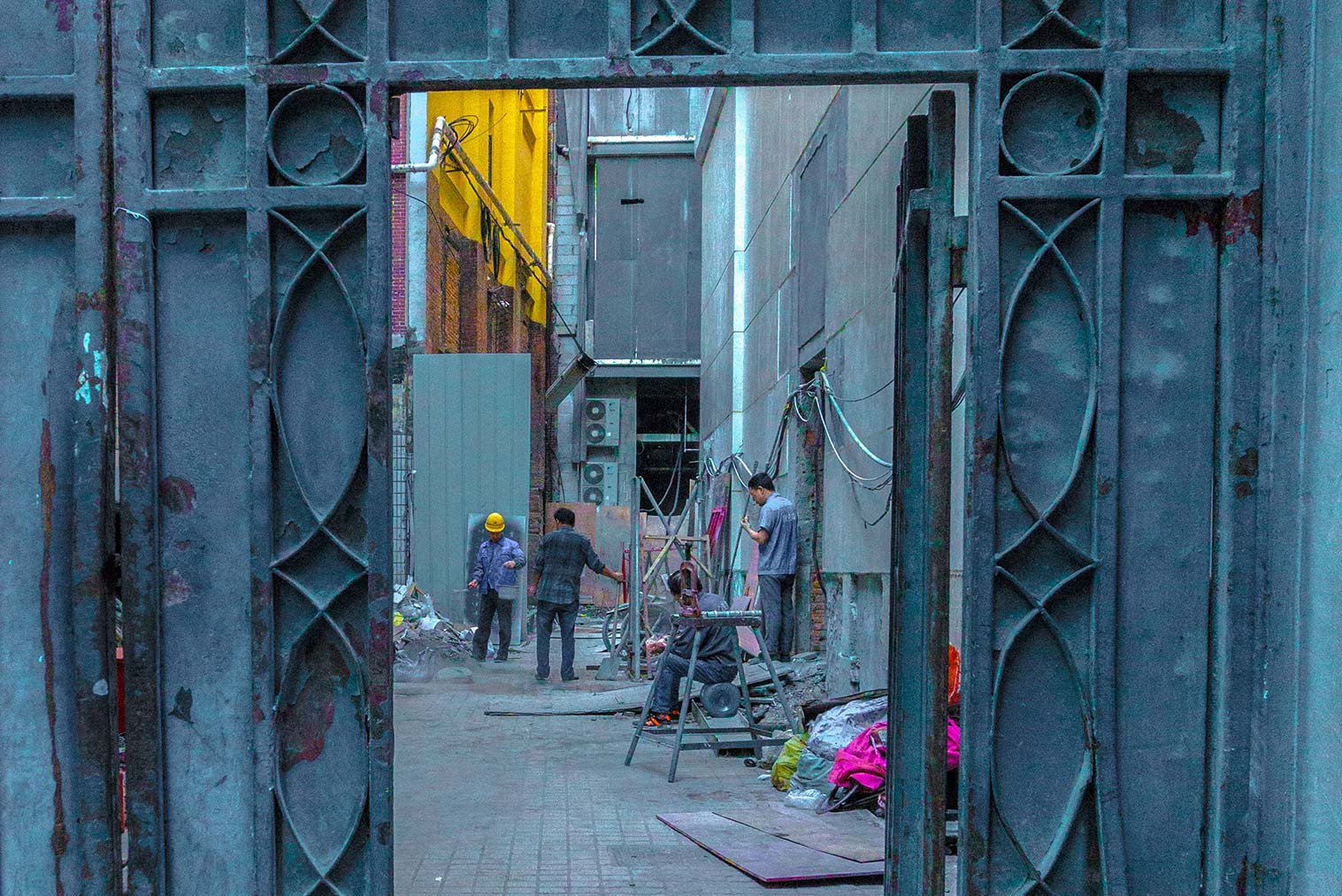
Paper’s contributions
This paper proposes new ways of determining which types of mobility measures are suitable for the purpose of empirically implementing conventional notions about the meaning of mobility comparisons. We derive a class of mobility indices that satisfy a minimal set of requirements for mobility comparisons, has a natural interpretation in terms of distributional analysis, and for which we develop reliable inference.
What makes our approach to mobility measurement novel is not the introduction of a particular new index, but rather a way of rethinking how to represent the problem, and therefore what theoretical and statistical treatment to apply to this representation of mobility. The key step involves a logical separation of fundamental concepts into (1) the measure of individual status, which could be income, position in the distribution, or something else, and (2) the aggregation of changes in status. The aggregation of changes in status involves applying standard principles to individual histories, which needs to be checked by the mobility measures.
Because our approach can capture income mobility and rank mobility within the same framework, it becomes possible to examine side-by-side mobility comparisons for each of the two underlying status concepts. As illustrated by an example from China around the millennium, this sheds light on the contrasting patterns of mobility through time, interpreted differently according to the type of mobility (up or down) and according to the status concept (income or rank). The use of our mobility measures shows that rank mobility decreased from pre- to post-millennium. By contrast, income mobility has carried on increasing; so has income inequality. An upward/downward decomposition for income-status shows that the story is mainly shaped by upward income movements that significantly increased between the two periods.
Research process
There is a large literature on inequality measurement. The standard theory of inequality measurement assumes that the equalisand is a cardinal quantity, with known cardinalisation. However, inequality comparisons often need to be made in situations where either the cardinalisation is unknown or the underlying data are categorical. In a previous paper, we proposed an alternative approach to inequality analysis that embeds both the ordinal-data problem and the well-known cardinal-data problem. We illustrated how it can be applied to the inequality of happiness and of health. In this paper, we extend the approach to mobility. The new class of mobility measures embeds both the cardinal case (income mobility) and the ordinal case (rank mobility), and it contains the wellknown class of generalized entropy inequality measures as a special case (when income distribution in period 1 gives the same amount to each individual).